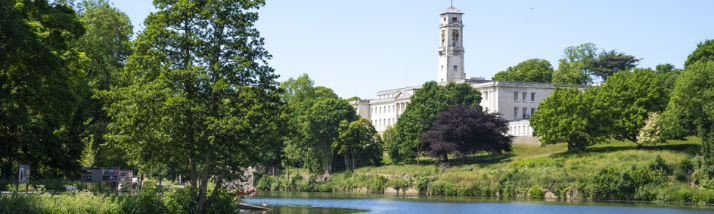
Deep machine learning to capture the performance of plants
Crop phenotyping performs a crucial role in the identification of higher-yielding plants, which in itself offers one solution to the continuing challenge of global food security. The current challenge for the field lies in capturing sufficient information to help determine how a plant is performing. The University of Nottingham’s Computer Vision Laboratory (CVL) is collaborating with Syngenta to explore the potential of convolutional neural net (CNN) architectures, a type of artificial deep neural network used in image analysis and computer vision, for plant feature extraction to aid improvement of products and processes in the agricultural and food industries.
Professor Tony Pridmore, Professor of Computer Science at the University of Nottingham, developed these deep learning techniques together with Drs Andrew French, Michael Pound and Aaron Jackson. CNNs are capable of detecting plant features, like leaf tips and wheat ears, in images. These features can be used to gather information on plant structure, function and performance which can then be applied to computational models to simulate plant behaviour in a range of environmental conditions.
A number of exploratory projects are underway, with a focus on detection and counting of biological objects. One of these has already provided a method capable of fast and accurate counting of seeds on sunflower heads, while others have considered images of wheat heads and insects on leaves e.g. to look for signs of pathogens or pests. Human counting by eye of many small objects lacks accuracy, and the values reported by different people are often not consistent. Therefore, the ability to perform objective, accurate counting allows a company like Syngenta to make the right choices between breeding materials and/or to select the most efficacious crop protection molecules to bring to market.
Artificial neural networks require a large number of annotated images to be effectively trained in image analysis. Access to a library of annotated images is a limiting factor for many researchers, denying them the opportunity to explore the use of such methods for their own projects. As part of their work, the CVL now helps to address this issue by developing and running the Annotated Crop Image Database, making the required training data available to the scientific community. The database currently hosts wheat, oilseed rape and Arabidopsis data, and there are planned improvements to enhance the user experience and support new types of data such as video, 3D and multi-view.